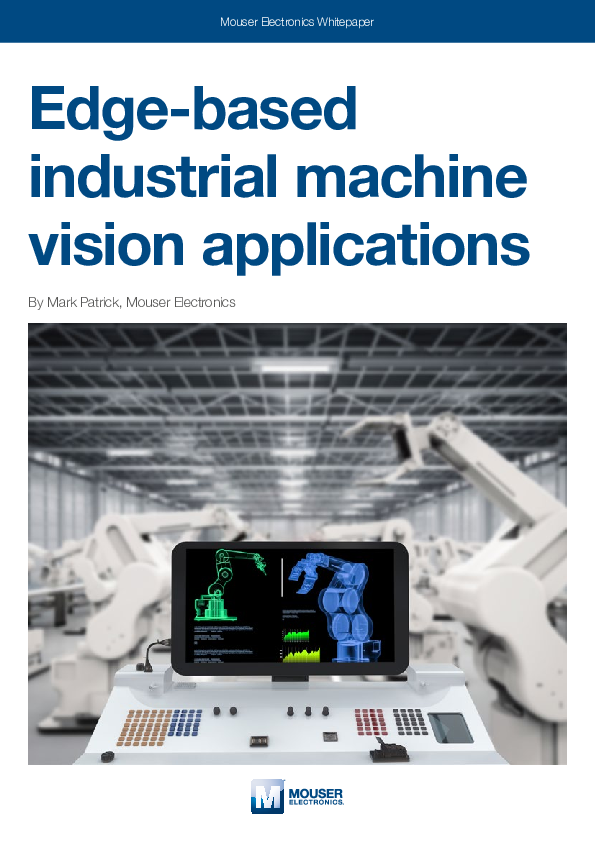
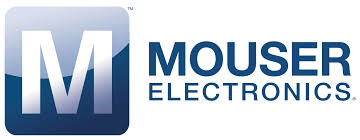
Edge-based industrial machine vision applications
Machine vision systems are pervasive. You’ll find them everywhere you go and they are part of everything you use. From spam filters to image recognition and processing, they have permeated our daily lives, whether at home, at work, or commuting between the two. Within the industrial manufacturing and production environments you’ll find many machine vision based systems deployed. The basic approach is that digital cameras capture images of, say, packets of rice being filled and sealed. In real time, computer algorithms are used to process the images and, by comparing them against known “good” images, detect problems. Perhaps the bag has split, or has not been filled completely, or maybe the sealing process has left a corner open. As machine vision has advanced, so has its ability to detect more and more potential failure conditions, resulting in improved production quality and throughput.
The algorithms used in such machine vision systems are broadly classified as artificial intelligence (AI) based and utilize deep-learning neural networks to digitally “see” the objects to be checked. As manufacturing operations continue to be transformed with initiatives such as Industry 4.0 and the Industrial Internet of Things (IIoT), machine vision applications will play an increasing role in improving operational efficiencies. Unlike early AI implementations, which required huge compute resources that were often constrained to a data center, today’s AI-based machine vision applications need to be closer to the “edge” in order to provide highly accurate, fast and actionable image detection.